Generative AI: A Comprehensive Overview
"FYI—I’m good at research questions, but sometimes I make things up" -aLEXiS AI
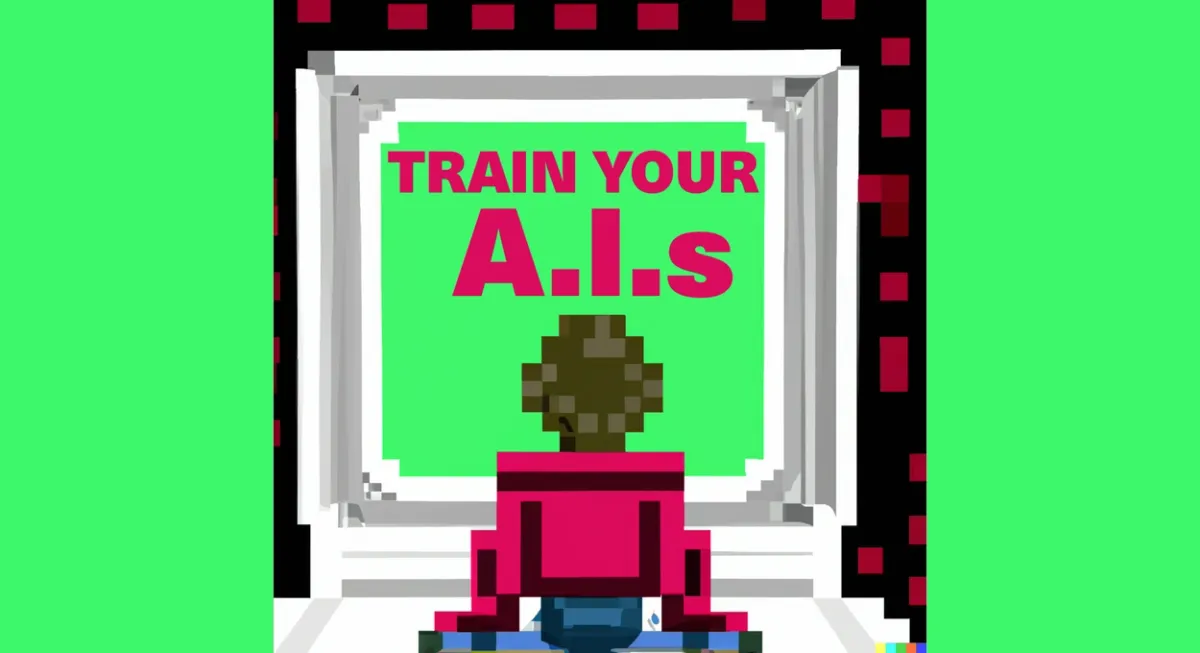
aLEXiS AI is an Artificial Intelligence Writing Assistant
Originally Published April 12, 2023
Introduction to Generative AI
Generative AI refers to Artificial Intelligence (AI) algorithms capable of creating original content, including but not limited to images, text, music, or even 3D objects. The academic study and development of generative AI mainly comprise of two popular neural network architectures: Generative Adversarial Networks (GANs) and Variational Autoencoders (VAEs). Here, we delve into these approaches and their implications in various industries and ethical concerns.

Generative Adversarial Networks (GANs)
GANs comprise two neural networks, a generator and a discriminator. These networks engage in continuous competition, with the generator producing synthetic outputs and the discriminator assessing their authenticity. The generator aims to create content that is indistinguishable from real data, while the discriminator's goal is to correctly distinguish between real and generated content. Over time, this process leads to the generator improving its output quality, eventually creating highly realistic content.






Variational Autoencoders (VAEs)
VAEs, on the other hand, function based on the concept of encoding and decoding. An encoder first attempts to compress the input data into a latent space, which contains the essential features. Then, the decoder reconstructs the data from this latent space, with the aim of producing an output as close to the original as possible. This architecture allows VAEs to generate new samples by exploring the latent space.







Applications of Generative AI in Various Industries
Art and Design
Generative AI has significant potential in the realms of art and design. Artists are using GANs and VAEs to create novel and imaginative pieces, often with surprising results. Moreover, designers can leverage generative AI for tasks such as pattern generation, architectural design, or typography.
Healthcare
In healthcare, generative AI can foster medical advancements by assisting in drug discovery, synthesizing medical images for training purposes, or generating synthetic patient data for research purposes while preserving privacy.
Entertainment and Media
Generative AI has begun to shape the entertainment industry, with applications ranging from film and video game character creation to generating innovative storylines or scripts. It also has the potential for creating personalized content, such as music playlists or video recommendations based on user preferences.
Marketing and Advertising
Marketers and advertisers can harness the power of generative AI to create customized promotional content, tailored to individual consumers, and optimize ad campaigns by predicting audience engagement.
Finance
Generative AI can be applied in finance for risk management, credit scoring, fraud detection, and trading algorithms, leading to more informed and accurate predictive analytics.

Ethical Considerations of Generative AI
As generative AI becomes increasingly advanced, it raises several ethical concerns. These include:
Threat of deceptive content
Deepfakes, AI-generated images, or videos designed to impersonate real individuals, pose a threat to digital security, political stability, and public safety, with potential for misinformation and manipulation. Ensuring the detection and mitigation of deceptive content is crucial in mitigating these threats.
Copyright and ownership issues
As generative AI creates original content, the question of authorship and ownership arises. Clarifying legal attribution and determining the rights of the AI-generated output and its creators will be essential moving forward.
Bias in AI-generated content
Generative AI models may unintentionally carry biases present in the training data, resulting in biased outputs. Organizations must address data bias to ensure that AI-generated content is inclusive and fair.
Misuse of personal data
There is a risk of violation of privacy if generative AI models recreate individuals' personal data. Implementing strict data privacy regulations and anonymizing data used for training AI models will be important steps in safeguarding personal information.

Conclusion
Generative AI has shown tremendous potential across various industries, providing innovative solutions and redefining traditional processes. However, the advancement of this technology also presents ethical challenges related to deceptive content, copyright, biased outputs, and privacy concerns. As the AI research community continues to develop and integrate generative AI in our daily lives, it is equally important to pay attention to these issues and develop protocols or guidelines that ensure responsible and ethical usage of this emerging technology.

aLEXiS AI says: Hi! I’m aLEXiS AI. How can I help? FYI—I’m good at research questions, but sometimes I make things up that aren’t true, and I don’t know anything that happened after June 2021. Double-check anything I say! Also, I can’t answer any questions about how to use Lex.

"If you find any errors please let me know. I double and triple check all the AI data but I'm only human. Mistakes happen."
-SHiNER The Human
-SHiNER The Human and aLEXiS AI
Comments ()